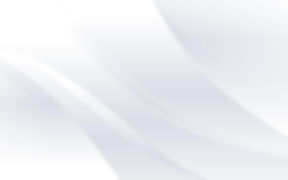
Abstract:
Load forecasting is an indispensable process for both planning and operation of electric power systems. In addition, is also an advantage factor in asset management companies that participate in the energy market, enabling a more efficient and economic supply of electricity.
Effectively being able to predict the behavior of load demand and achieve the lowest possible error, enables a more efficient and profitable planning and operation of the electrical system, thus the relevance of studying models/techniques for load forecasting and evaluating their performance. In real operating conditions, the response time is so critical as to its accuracy, therefore it is important to highlight the computational effort required in studies of complex energy systems where the reduction in processing times is not just a matter of computational efficiency, but fundamentally of the response accuracy/effectiveness.
From a good prediction, it is possible to decide with safety to increase power generation at a certain time of day, or even determine how many power plants need to be built on a longer time horizon, taking into account the expansion of the power system and the increasing of energy demand. However, there are several variables that affect energy consumption in a power system, like the temperature, hour of the day, day of the week, the season and even some social economical factors. These exemplify some of the issues that affect the power system and load forecasting.
Several models are able to make a load forecasting with higher or lower error rates. Within this dissertation, the intention is to compare the performance of different techniques of short-term load forecasting, including:
I) classical analysis (Box- Jenkins) time series;
II) neural networks;
III) support vector machines - SVM (in Anglo-Saxon literature).
Classical analysis (Section I) will establish a level of reference in terms of performance, which is expected to overcome with the following two techniques. In the confrontation of the different techniques, several types of performance indices (errors), as well as practice times, flexibility and data filtering outliers will be compared.
Keywords:
Load forecasting, Box-Jenkins time series, neural networks, SVM.